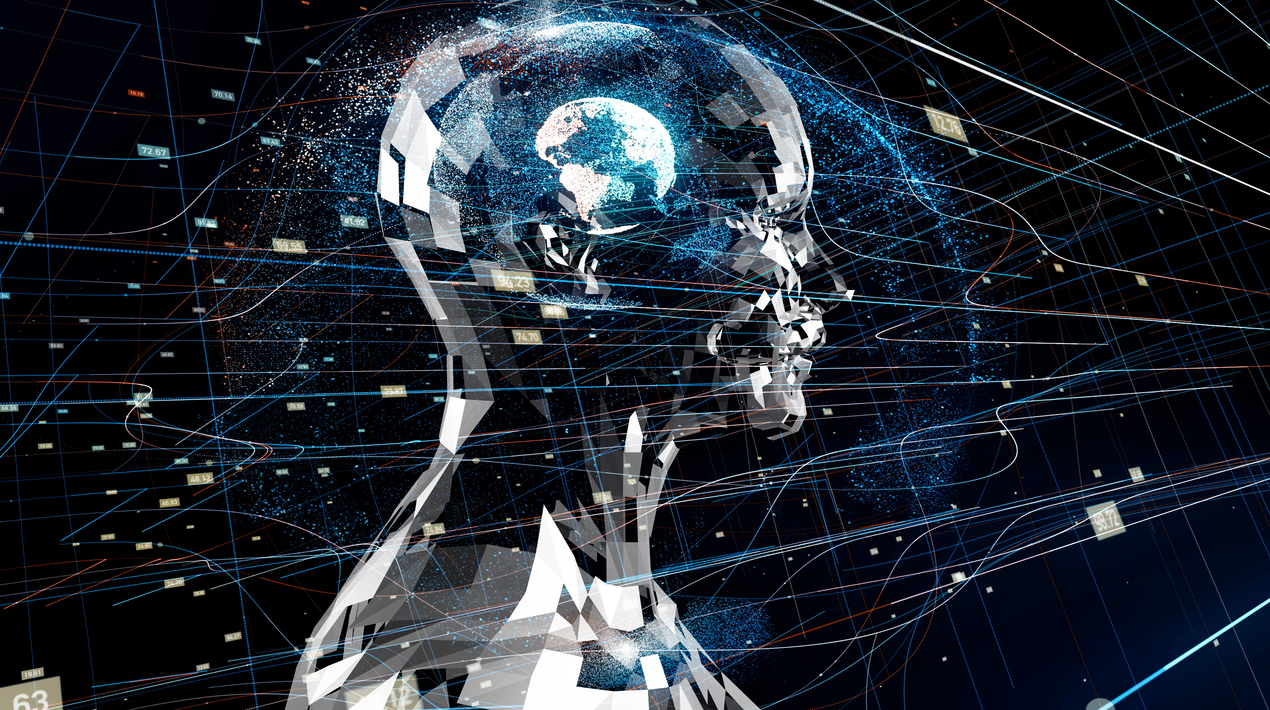
When hiring, many organisations use artificial intelligence tools to scan resumes and predict job-relevant skills. Colleges and universities use AI to automatically score essays, process transcripts and review extracurricular activities to predetermine who is likely to be a good student. In response to claims of unfairness and bias in tools used in hiring, college admissions, predictive policing, health interventions, and more, the University of Minnesota recently developed a new set of auditing guidelines for AI tools.
The auditing guidelines, published in the American Psychologist, were developed by Richard Landers, associate professor of psychology at the University of Minnesota, and Tara Behrend from Purdue University. They apply a century’s worth of research and professional standards for measuring personal characteristics by psychology and education researchers to ensure the fairness of AI.
The researchers developed guidelines for AI auditing by first considering the ideas of fairness and bias through three major lenses of focus:
- How individuals decide if a decision was fair and unbiased
- How societal, legal, ethical and moral standards present fairness and bias
- How individual technical domains—like computer science, statistics and psychology—define fairness and bias internally
Using these lenses, the researchers presented psychological audits as a standardised approach for evaluating the fairness and bias of AI systems that make predictions about humans across high-stakes application areas, such as hiring and college admissions.
There are twelve components to the auditing framework across three categories that include:
- Components related to the creation of, processing done by and predictions created by the AI
- Components related to how the AI is used, who its decisions affect and why
- Components related to overarching challenges: the cultural context in which the AI is used, respect for the people affected by it and the scientific integrity of the research used by AI purveyors to support their claims
The researchers recommend the standards they developed to be followed both by internal auditors during the development of high-stakes predictive AI technologies, and afterwards by independent external auditors. Any system that claims to make meaningful recommendations about how people should be treated should be evaluated within this framework.
Industrial psychologists have unique expertise in the evaluation of high-stakes assessments. Their goal was to educate the developers and users of AI-based assessments about existing requirements for fairness and effectiveness and to guide the development of future policies that will protect workers and applicants.
AI models are developing so rapidly, it can be difficult to keep up with the most appropriate way to audit a particular kind of AI system. The researchers hope to develop more precise standards for specific use cases, partner with other organisations globally interested in establishing auditing as a default approach in these situations and work toward a better future with AI more broadly.
As reported by OpenGov Asia, creating smarter, more accurate systems requires a hybrid human-machine approach, according to researchers at the University of California, Irvine. In a study published this month in Proceedings of the National Academy of Sciences, they present a new mathematical model that can improve performance by combining human and algorithmic predictions and confidence scores.
To test the framework, researchers conducted an image classification experiment in which human participants and computer algorithms worked separately to correctly identify distorted pictures of animals and everyday items—chairs, bottles, bicycles, trucks. The human participants ranked their confidence in the accuracy of each image identification as low, medium or high, while the machine classifier generated a continuous score. The results showed large differences in confidence between humans and AI algorithms across images.
This interdisciplinary project was facilitated by the Irvine Initiative in AI, Law, and Society. The convergence of cognitive sciences—which are focused on understanding how humans think and behave—with computer science—in which technologies are produced—will provide further insight into how humans and machines can collaborate to build more accurate artificially intelligent systems.