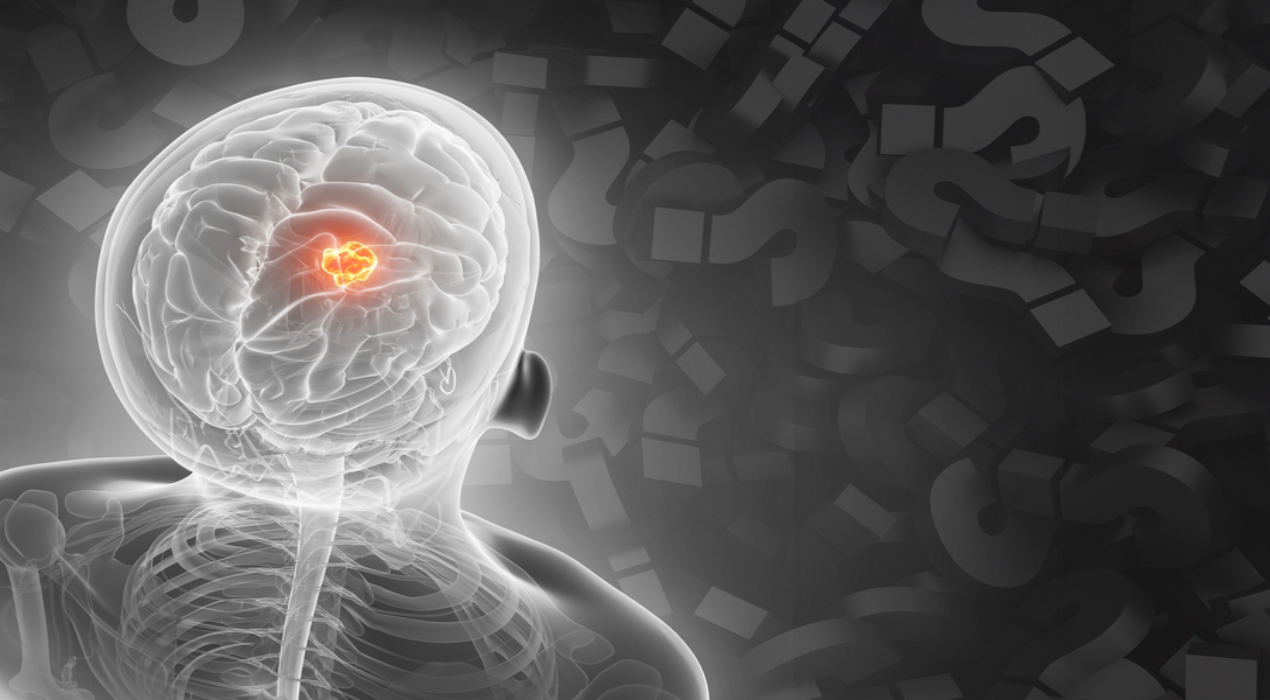
Getting your Trinity Audio player ready...
|
A novel study conducted by scientists from MIT and one of the cancer institutes has introduced a method to simplify the identification of the sources of enigmatic cancers. Through the application of machine learning, the researchers devised a computational framework capable of analysing the genetic sequences of approximately 400 genes. This framework can then predict the primary location in the body from which a particular tumour originated.
“In a minority of cancer cases, a subset of patients faces the challenge of having an unidentified source for their cancer. This circumstance considerably complicates the selection of appropriate treatments, as numerous cancer medications are customarily designed to address types of cancer,” said Intae Moon, an MIT graduate student in electrical engineering and computer science who is the lead author of the new study.
By utilising this framework, the researchers successfully demonstrated their ability to categorise at least 40% of unidentified origin tumours with a high level of confidence. This accomplishment was evident in a dataset comprising around 900 patients. By employing this approach, the researchers enabled a 2.2-fold increase in the number of patients who could have been eligible for precision-targeted treatment based on the genomic origin of their cancer.
In approximately 3 to 5 % of individuals who have cancer, especially instances involving the spread of tumours across the body, medical professionals encounter challenges in ascertaining the original site of cancer origin. Such tumours are categorised as cancers of unknown primary (CUP).
The absence of this information frequently hinders physicians from administering “precision” medications to patients. These medications are usually authorised for well-defined cancer categories in which their efficacy has been established. These focused treatments typically exhibit superior effectiveness and fewer adverse effects than treatments designed for various cancers commonly prescribed to individuals with CUP.
Moon, an associate linked to the Computer Science and Artificial Intelligence Laboratory, chose to examine routinely collected genetic data to forecast cancer types. This genetic data involves sequences from about 400 genes frequently subject to cancer mutations. The researchers employed a machine-learning model trained on data from nearly 30,000 patients diagnosed with one of the 22 recognised cancer types.
To validate the model’s accuracy, researchers compared CUP patients’ survival duration with the projected prognosis based on the model’s cancer type prediction. A pattern emerged: patients predicted to have grim-prognosis cancers, like pancreatic cancer, had shorter survival; those with more favourable prognosis types, like neuroendocrine tumours, had more prolonged survival.
The model’s value was underscored by treatment analysis. About 10% of CUP patients received targeted treatments guided by oncologists’ estimates of cancer origin. Aligning treatment with the model’s predicted cancer type yielded better outcomes than mismatched treatments.
Furthermore, through the model’s utilisation, the researchers identified an additional 15% of patients (reflecting a 2.2-fold increase) who could have been suitable candidates for existing targeted treatments had their cancer type been known. Instead, these patients had been administered more generalised chemotherapy drugs.
The researchers are enthusiastic about broadening their model’s scope to encompass additional forms of data, including pathology images and radiology images. This extension offers a more all-encompassing prediction by incorporating multiple data modalities. By doing so, the model could acquire a comprehensive understanding of tumours, allowing it to predict the tumour type and patient prognosis and conceivably even the most suitable treatment approach.