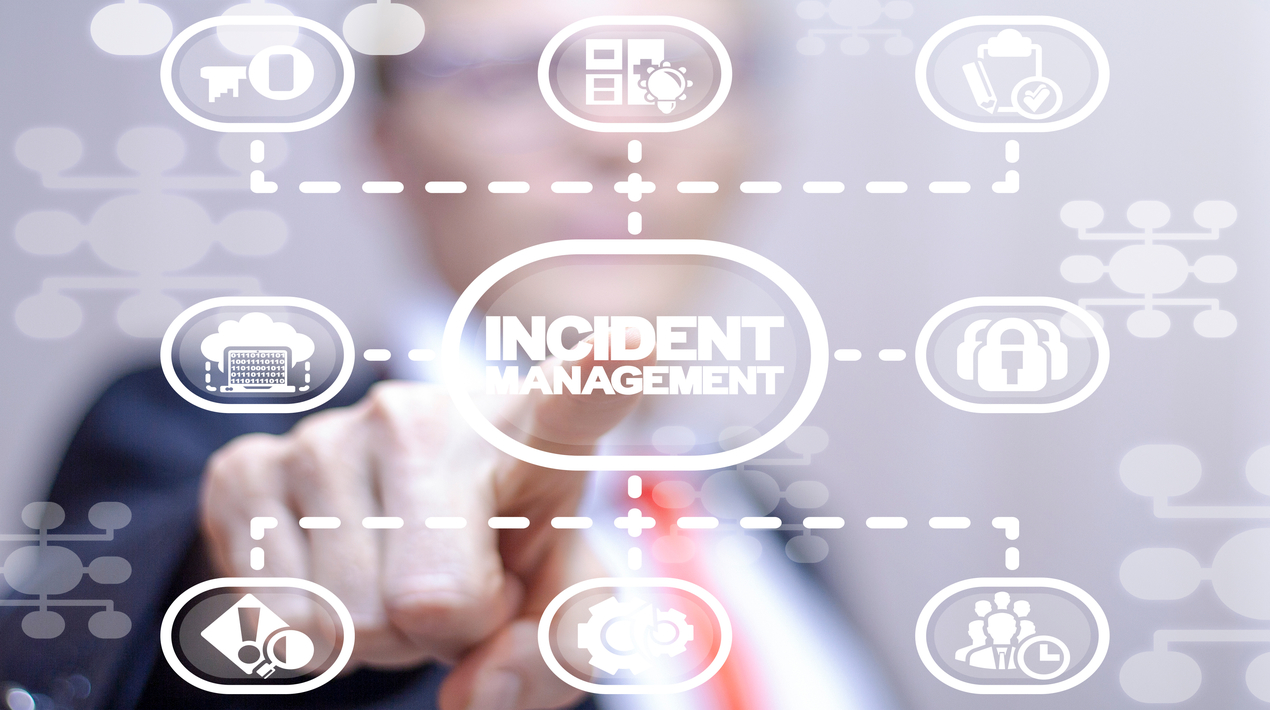
U.S Researchers from the National Science Foundation’s SimCenter built the Building Recognition using AI at Large-Scale (BRAILS) suite of tools. BRAILS is an AI-enabled software to assist regional-scale simulations that extracts information from satellite and street view images for being used in computational modelling and risk assessment of the built environment.
BRAILS creates enhanced building databases for cities by running artificial intelligence-powered simulations on high-performance computers at the Texas Advanced Computing Center (TACC). The researcher says that they want to simulate the impact of hazards on all of the buildings in a region, but they don’t have a description of the building attributes. Therefore, they use AI to get the needed information. They can train neural network models to infer building information from images and other sources of data.
The researchers have two objectives which are damage mitigation and real scenario simulation. First, they mitigate the damage in the future by doing simulations and providing results to decision and policy-makers. Second, they use this data to quickly simulate a real scenario, instantly following a new event, before the reconnaissance team is deployed. They aim to provide near-real-time simulation results that can help guide emergency response with greater accuracy.
The basic BRAILS framework uses computer vision to automatically pull building details – architectural features such as roofs, windows, and chimneys — from satellite and ground-level images found in Google Maps and merges these with other datasets, such as Microsoft Footprint Data and OpenStreetMap. BRAILS users can also enhance the data with tax records, city surveys and other information to get more accurate assessments.
A crowdsourcing effort has contributed to some of the data labelling. Volunteers with the SimCenter’s Building Detective for Disaster Preparedness project identified specific architectural features of structures, such as garages, roofs and adjacent buildings. They use the data to train additional machine learning modules. The citizen science project was launched in March. Within a few weeks, a thousand volunteers had annotated 20,000 images.
The researchers ran a series of testbeds to determine the accuracy of the AI-based models. Each generated an inventory of a city’s structures and simulated the impact of a hazard-based on historical or plausible events. The team has created testbeds for earthquakes in San Francisco, and hurricanes in Texas and New Jersey.
To train the BRAILS modules and run the simulations, the researchers relied on TACC’s supercomputers, the fastest academic supercomputer in the world, and Maverick 2, a GPU-based system designed for deep learning.
The hazard event simulations such as applying wind fields or ground shaking to thousands or millions of buildings to assess the impact of a hurricane or earthquake require a lot of computing resources and time. For one city-wide simulation, depending on the size, it typically takes hours to run on TACC. Regarding the performance of BRAILS, the accuracy is close to 100% for some models like occupancy. For other modules like roof type, the accuracy is about 90%.
The U.S. has been utilising technology for disaster mitigation, such as developing an Earthquake early-warning system. As reported by OpenGov Asia, The Earthquake early-warning system called ShakeAlert is now available to residents of California, Oregon and Washington after 15 years of planning and development.
It reaches 50 million people in the most earthquake-prone region in the U.S. People in these three states can now receive alerts from a wireless emergency alert system, third-party phone apps, and other technologies. Hence, the system will give them precious seconds of warning before an earthquake hits. The ShakeAlert system aims to facilitate the delivery of public alerts of potentially damaging earthquakes and provide warning parameter data to government agencies and private users on a region-by-region basis.