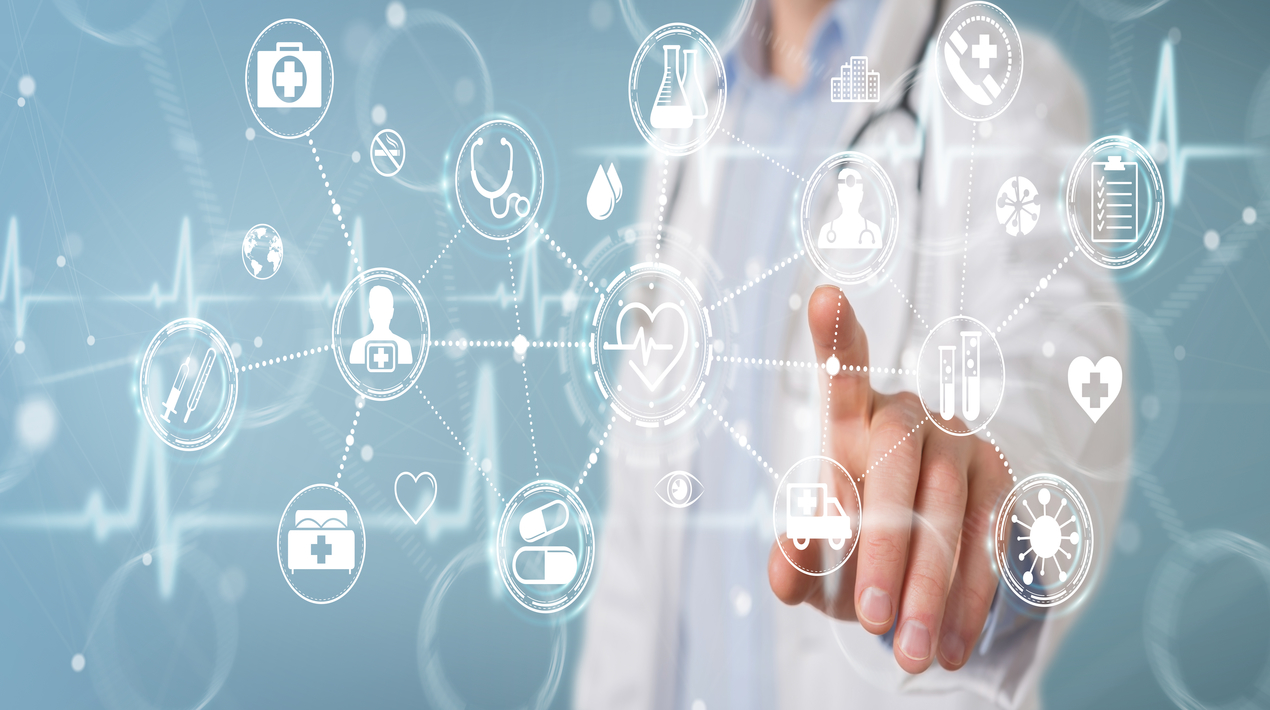
A new study by the Oregon State University College of Engineering shows that machine learning techniques can offer powerful new tools for advancing personalised medicine, care that optimises outcomes for individual patients based on unique aspects of their biology and disease features.
The research with machine learning, a branch of Artificial Intelligence (AI) in which computer systems use algorithms and statistical models to look for trends in data, tackles long-unsolvable problems in biological systems at the cellular level.
Those systems tend to have high complexity—first, because of the vast number of individual cells and second, because of the highly nonlinear way in which cells can behave. Nonlinear systems present a challenge for upscaling methods, which is the primary means by which researchers can accurately model biological systems at the larger scales that are often the most relevant.
The new research, funded in part by the U.S. Department of Energy and published in the Journal of Computational Physics, is one of the first examples of using machine learning to address issues with modelling nonlinear systems and understanding complex processes that might occur in human tissues.
The advent of machine learning has given us a new tool in our arsenal to solve problems we could not solve before. While the tools themselves are not necessarily new, the particular applications we have are very different. We are beginning to apply machine learning in a more constrained way, and this is allowing us to solve physical problems we had no way of solving before.
– Professor, Environmental Engineering, Oregon State University
In modelling cellular activity within an organ, it is not possible to individually model each cell in that organ—a cubic centimetre of tissue may contain a billion cells—so researchers rely on what’s known as upscaling.
Upscaling seeks to decrease the data required to analyze or model a particular biological process while maintaining the fidelity—the degree to which a model accurately reproduces something—of the core biology, chemistry and physics occurring at the cellular level.
By reducing the information load for a very complicated system at the cellular level, researchers can better analyse and model the impact or response of those cells with high fidelity without having to model each individual one. The researchers describe it as simplifying a computational problem that has tens of millions of data points by reducing it to thousands of data points.
The new approach could pave the way to potential patient treatments based on numerical model outcomes. In this study, researchers were able to employ machine learning and develop a novel method to resolve classic nonlinear problems in biological and chemical systems.
The promises of individualised medicine are rapidly becoming a reality. The combination of multiple disciplines—such as molecular biology, applied mathematics and continuum mechanics—are being combined in new ways to make this possible. One of the key components of this will certainly be the continuing advances in machine learning methods.
U.S. researchers have been utilising machine learning in various areas, including healthcare. As reported by OpenGov Asia, to help clinicians avoid remedies that may potentially contribute to a patient’s death, researchers at MIT have developed a machine learning model that could be used to identify treatments that pose a higher risk than other options. Their model can also warn doctors when a septic patient is approaching a medical dead end — the point when the patient will most likely die no matter what treatment is used — so that they can intervene before it is too late.
When utilised to a dataset of sepsis sufferers in a hospital intensive care unit, the investigator mannequin confirmed that about 12% of the therapies for deceased sufferers have been dangerous. The research additionally exhibits that about 3 p.c of sufferers who didn’t survive have been caught in a medical stalemate 48 hours earlier than demise.