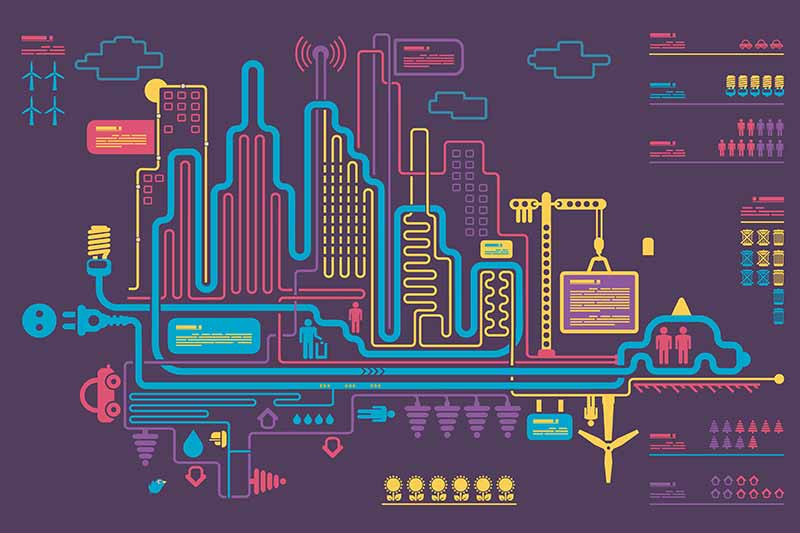
It has been highlighted over the past few months that in cities, outbreaks or clusters of COVID-19 positive individuals can grow very fast in heavily populated built up areas.
Tracking how people move around urban areas can pinpoint where disease might transmit fastest and farthest.
Places where there have been large gatherings of people have seen high infection rates such as a few notable Church gatherings, outbreaks from people socialising in nightclubs and restaurants as well as outbreaks in residential blocks.
Governments have the task of predicting where are the places that have the highest probability of spreading the disease and governments need to be equipped with the tools and technology to help them do this.
Big-data studies of human mobility need to be combined with epidemiological models. And the demographic profiles of people coming into contact at any particular location need to be included.
In many cities, the details of everyday interactions in cities are not documented well enough to model risk factors accurately, as experiences with COVID-19 show. Resorts, conferences, religious gatherings and workplaces have all experienced notable outbreaks.
Groups living in close proximity are a very high risk risk. Almost 93% of Singapore’s COVID-19 cases in the first 48 days occurred in dormitories for migrant workers.
Each block houses hundreds or thousands of workers. Cases there increased rapidly in a very short space of time to more than 40,000, or more than 12% of that population, compared with fewer than 2,600 infections elsewhere in the city-state of 5.3 million people.
Mapping the Spread of COVID-19
A model of disease spread can be built and refined as data and knowledge improve on human flows on three levels. City-wide – a map which highlights the main flows of people throughout the city. Then by busiest locations and busiest timings should then be mapped and then thirdly record demographics and types of human interactions.
By combining all these insights, governments will be better able to anticipate superspreading locations and target precautionary measures, such as delaying reopening businesses, quarantining arrivals, tightening crowd control and intensifying cleaning and disinfection in particular places.
How Government can tap into pre-existing resources
All sources of data on human mobility need to be tapped. For example, ‘smart’ cities such as Singapore have networks of cameras on lamp posts to track traffic flows. These could be reconfigured to track the density and mixing of people anonymously.
Data from geolocation and contact-tracing apps can map where people go, who they interact with and for what length of time.
Funding agencies can help fund studies of human movement and interactions in key superspreading locations such as transport hubs.
Urban analysts and modellers need to study and document the types of face-to-face interactions, networks and crowd mixing.
Governments should use these data and models to target their public-health strategies. More effective targeting of measures will help to avoid ‘virus fatigue’ among the public and help education and the economy by allowing places to minimize the risks of some kinds of reopening.