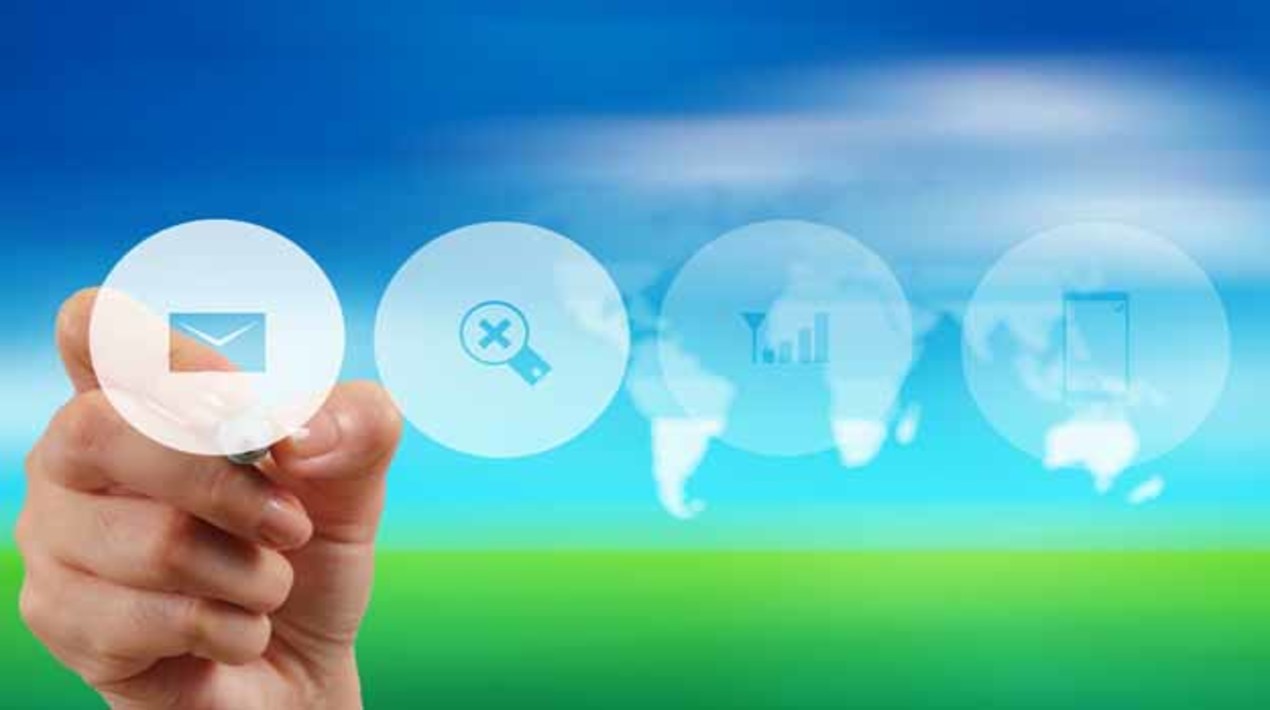
With this new spam detection and filtering system, the aim is to achieve greater precision and quicker results so that this type of email does not “sneak” into our inboxes. Unwanted email messages, or spam messages, are those messages that we receive from unknown senders and that not only fill our inbox with junk, but could also hide the occasional link to a fraudulent site or even malware.
Although the current filters that use email clients usually work quite well, they always end up sneaking a series of spam emails into our inbox, which sometimes out of laziness, people leave them there, something that could even end up affecting the limited storage of these email clients. This technique, presented in a paper published in the International Journal of Intelligent Robotics and Applications, could help to improve the security of users, while also helping them to skim irrelevant or undesired emails.
Although the automatic filtering of these emails and the identification of spam messages has come a long way, now researchers have created a new technique for the automatic detection of spam emails that could help improve the security of users and prevent them from receiving these types of messages. Unlike other models, it increases the convergence rate of spam detection, achieving better results. The model also reduces training speeds and leads to higher sorting efficiency.
This model relies on multi-objective feature selection under a new and promising deep learning technique. And it is that unlike other methods developed before, the model is delivered in a set of image and text data. The work confirms the promise of new and improved detection models based on deep learning algorithms. Our model introduces a new hybrid heuristic algorithm and achieves optimal feature selection with a multi-objective function.
In this way, it is a new model that is easy to implement and that, above all, can be delivered quickly in short periods of time, unlike current models that require the participation of a larger group of people. The model could improve the quality of life for people who receive a lot of emails by allowing them to navigate through their mail seamlessly and only use their accounts for their intended purpose.
In the future they want to implement this new filtering technique on a large scale, improving the security and efficiency of current email services. The security of spam detection and filtering systems is crucial to achieving greater accuracy and reliable results that can be improved in the future through joint learning. The false-positive rate of many models is still higher than required but should be reduced to the smallest possible value in the future.
As reported by OpenGov Asia, creating smarter, more accurate systems requires a hybrid human-machine approach, according to researchers at the University of California, Irvine. In a study published this month in Proceedings of the National Academy of Sciences, they present a new mathematical model that can improve performance by combining human and algorithmic predictions and confidence scores.
To test the framework, researchers conducted an image classification experiment in which human participants and computer algorithms worked separately to correctly identify distorted pictures of animals and everyday items—chairs, bottles, bicycles, trucks. The human participants ranked their confidence in the accuracy of each image identification as low, medium or high, while the machine classifier generated a continuous score. The results showed large differences in confidence between humans and AI algorithms across images.
This interdisciplinary project was facilitated by the Irvine Initiative in AI, Law, and Society. The convergence of cognitive sciences—which are focused on understanding how humans think and behave—with computer science—in which technologies are produced—will provide further insight into how humans and machines can collaborate to build more accurate artificially intelligent systems.