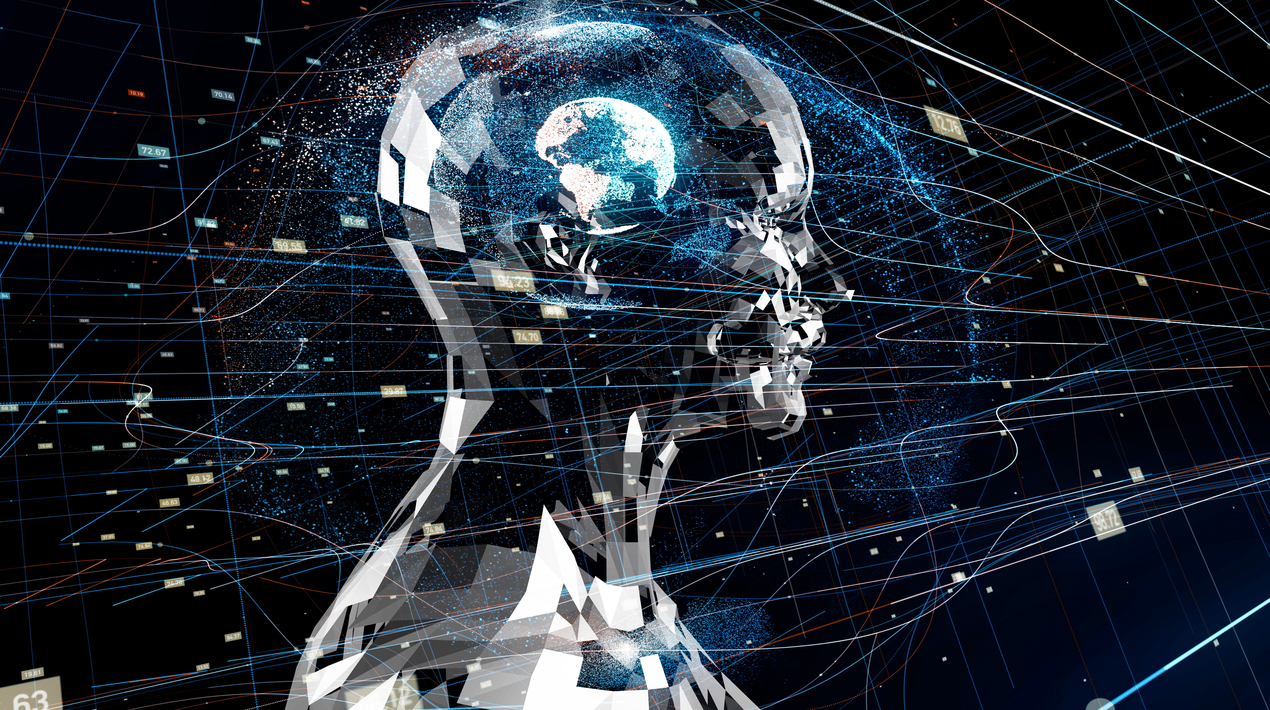
Federated learning is a great tool for training Artificial Intelligence (AI) systems while protecting data privacy, but the amount of data traffic involved has made it unwieldy for systems that include wireless devices. A new technique uses compression to drastically reduce the size of data transmissions, creating additional opportunities for AI training on wireless technologies.
Federated learning is a form of machine learning involving multiple devices, called clients. Each of the clients is trained using different data and develops its own model for performing a specific task. The clients then send their models to a centralised server.
The centralised server draws on each of those models to create a hybrid model, which performs better than any of the other models on their own. The central server then sends this hybrid model back to each of the clients. The entire process is then repeated, with each iteration leading to model updates that ultimately improve the system’s performance.
One of the advantages of federated learning is that it can allow the overall AI system to improve its performance without compromising the privacy of the data being used to train the system. For example, you could draw on privileged patient data from multiple hospitals in order to improve diagnostic AI tools, without the hospitals having access to data on each other’s patients
– Chau-Wai Wong, Assistant Professor, Electrical and Computer Engineering, North Carolina State University
There are many tasks that could be improved by drawing on data stored on people’s personal devices, such as smartphones. And federated learning would be a way to make use of that data without compromising anyone’s privacy.
However, there’s a stumbling block: federated learning requires a lot of communication between the clients and the central server during training, as they send model updates back and forth. In areas where there is limited bandwidth, or where there is a significant amount of data traffic, the communication between clients and the centralised server can clog wireless connections, making the process slow.
Specifically, the researchers developed a technique that allows the clients to compress data into much smaller packets. The packets are condensed before being sent and then reconstructed by the centralised server. The process is made possible by a series of algorithms developed by the research team. Using the technique, the researchers were able to condense the amount of wireless data shipped from the clients by as much as 99%. Data sent from the server to the clients is not compressed.
The technique makes federated learning viable for wireless devices where there is limited available bandwidth. For example, it could be used to improve the performance of many AI programs that interface with users, such as voice-activated virtual assistants.
As reported by OpenGov Asia, a new report showed that Artificial Intelligence (AI) has reached a critical turning point in its evolution. Substantial advances in language processing, computer vision and pattern recognition mean that AI is touching people’s lives daily—from helping people to choose a movie to aid in medical diagnoses.
With that success, however, comes a renewed urgency to understand and mitigate the risks and downsides of AI-driven systems, such as algorithmic discrimination or the use of AI for deliberate deception. Computer scientists must work with experts in the social sciences and law to assure that the pitfalls of AI are minimised.
In terms of AI advances, the panel noted substantial progress across subfields of AI, including speech and language processing, computer vision and other areas. Much of this progress has been driven by advances in machine learning techniques, particularly deep learning systems, which have leapt in recent years from the academic setting to everyday applications.