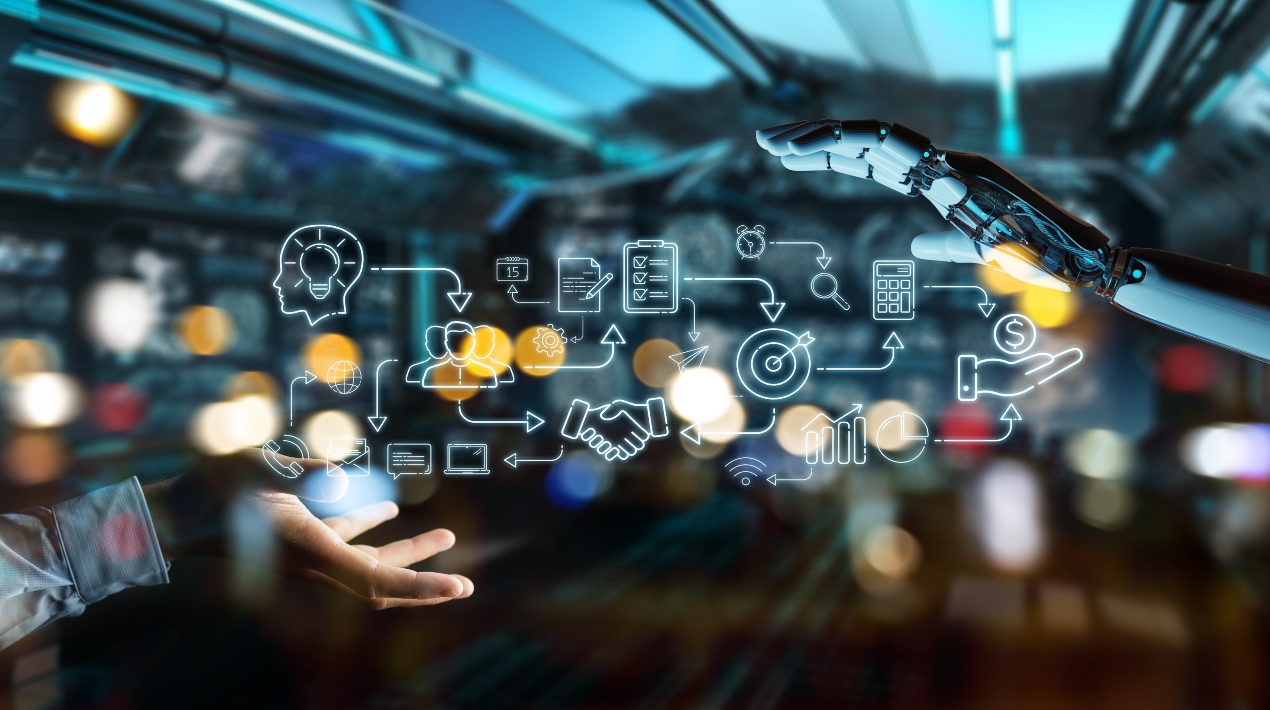
U.S. researchers have developed and tested the Explanation Methods also known as Interpretability Methods to try and explain how black-box machine learning models make predictions. At their most fundamental level, explanation methods are either global or local. While global explanations aim to describe an entire model’s overall behaviour, local explanation methods concentrate on explaining how the model arrived at a single prediction.
This is frequently accomplished by creating a different, more basic model that resembles the larger, black-box model. But because deep learning models function in fundamentally nonlinear and complex ways, it is particularly difficult to create a global explanation model that is effective.
According to Yilun Zhou, a graduate student in the Interactive Robotics Group of the Computer Science and Artificial Intelligence Laboratory (CSAIL), who studies models, algorithms, and evaluations in interpretable machine learning, this has caused researchers to shift much of their recent focus instead toward local explanation methods.
There are three major groups into which the most common local explanation techniques can be divided. The earliest and most widely used type of explanation technique is known as feature attribution. The features that the model prioritised when it made a certain decision are displayed through feature attribution methods.
A machine-learning model uses features as its input variables, which are then used to make predictions. In essence, feature attribution techniques reveal what the model concentrates on when making a prediction.
A counterfactual explanation is the second category of explanation technique. These techniques demonstrate how to alter an input such that it belongs to a different class given the input and the forecast of a model.
For instance, the counterfactual explanation reveals what must happen for a borrower’s loan application to be approved if a machine-learning algorithm predicts that the person will be denied credit. Perhaps the person must have a higher credit score or income, two factors that the algorithm utilised to make its prediction, to be accepted.
The third category of explanation approaches includes examples of important explanations. This approach needs access to the data that were used to train the model, unlike the others.
A sample importance explanation will highlight the training sample that a model most frequently used to make a certain prediction; ideally, this sample will be the one that is closest to the input data. This kind of justification is especially helpful when one notices an apparent irrationality in a prediction.
A specific sample that was used to train the model may have been impacted by a data entry error. With this knowledge, the sample might be fixed, and the model retrained for more precise operation.
End-users should exercise caution when attempting to employ explanation methods in practice, even if machine-learning practitioners may find them helpful occasionally when trying to find defects in their models or comprehend how a system operates.
Researchers assert that the community should put more effort into understanding how information is presented to decision-makers so they can grasp it. They also added that greater regulation is required to ensure machine-learning models are utilised responsibly in practice. The solution goes beyond better explaining techniques alone.
Researchers anticipate future studies on explanation approaches for specific use cases, such as model debugging, scientific discovery, fairness audits, and safety assurance, in addition to new work aimed at improving explanations.
By figuring out the fine-grained features of explanation methods and the needs of different use cases, researchers could come up with a theory that would match explanations to specific situations. This could help avoid some of the problems that come up when using explanations in the real world.