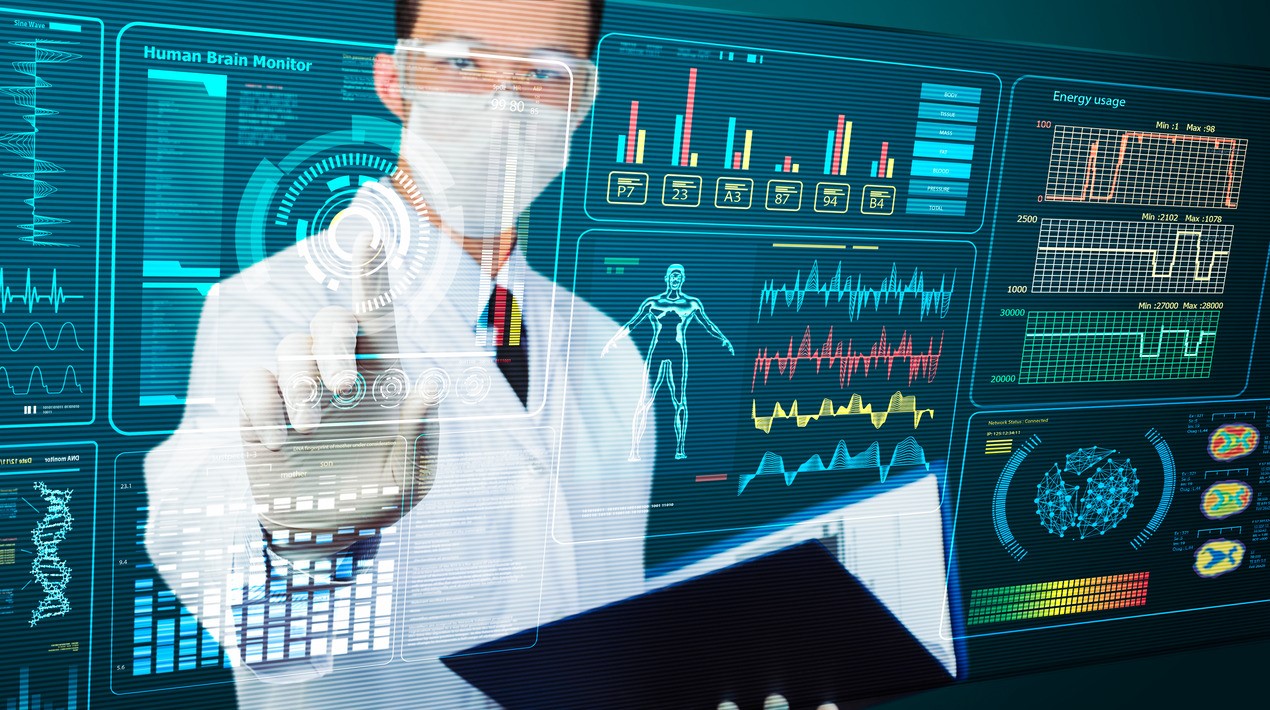
Getting your Trinity Audio player ready...
|
At the forefront of cutting-edge advancements, the Cancer Science Institute of Singapore (CSI Singapore) at the National University of Singapore (NUS) has pioneered an innovative AI-based methodology, revolutionising the accuracy of RNA 3D structure prediction.
This novel approach marks a significant leap forward in computational biology, offering a promising avenue for more precise modelling of RNA’s intricate atomic-level architectures.
Led by Professor ZHANG Yang, their pioneering AI-driven technique, DRfold, has revolutionised the modelling of atomic-level RNA 3D structures from primary RNA sequences, boasting a remarkable 70% improvement in accuracy compared to traditional methodologies.
Published in Nature Communications on September 16, 2023, their work signifies a significant advancement in understanding RNA, pivotal biomolecules crucial in transcription, translation, and the regulation of biological processes, positioning them as promising targets for novel therapeutics.
RNAs, single chains of nucleotides derived from DNA, have garnered attention for their potential in drug discovery. Unlike traditional protein-focused approaches, targeting RNAs with small molecules expands the horizon of drug design exponentially, intensifying academic and industry interest worldwide.
Traditionally, predicting RNA structures has been challenging due to their less stable folds and limited availability of experimental data. Physics- and statistics-based models struggle to capture the intricate folding interactions of RNAs accurately. Enter DRfold, introducing two innovative deep-learning network pipelines: one for end-to-end learning and the other for geometrical restraint learning. This approach significantly enhances the accuracy of AI-based force fields by leveraging self-attention transformer networks to predict 3D structures from RNA sequences.
Dr LI Yang, a key researcher, underscores the importance of determining RNA structures for function annotation and drug discovery. With traditional methods applicable to only a tiny fraction of known RNAs, their aim is to develop computational methods capable of predicting high-quality RNA structure models, addressing the substantial information gap.
The implications of this research in drug design and virtual screening are profound. Prof. Zhang emphasises how DRfold models can guide RNA drug design, optimise vaccine stability (such as mRNA vaccines), study biological functions of RNAs, and design novel RNA experiments. The open-source framework of DRfold facilitates its scalability and applicability for RNA-protein interaction modelling and other related problems.
Looking ahead, the team seeks to expand their AI strategy to predict protein-RNA interactions, an area currently lacking reliable AI approaches. They also aim to enhance DRfold’s accuracy in single-chain RNA structure prediction, especially for larger RNAs, by exploring innovative strategies to overcome the limitations posed by limited experimental data.
The strides made by CSI Singapore’s research team with DRfold not only offer a transformative approach to RNA structure prediction but also open doors to a new era of RNA-based therapeutics and biological research. The potential impact of their work extends across multiple domains, promising exciting advancements in the realms of medicine and molecular biology.
OpenGov’s recent coverage spotlighted the challenges in understanding cell-mediated cytotoxicity due to analysis limitations, highlighting Assistant Professor Cheow Lih Feng and his team’s groundbreaking solution at the NUS Institute for Health Innovation & Technology.
AI’s integration into healthcare and medicine heralds a transformative era, fundamentally altering diagnostics, treatment personalisation, and patient care. Its prowess in image analysis enhances diagnostic accuracy, predictive analytics aid in preemptive interventions, and tailored treatment plans optimise patient outcomes.
From expediting drug discovery to enabling remote monitoring and operational streamlining, AI’s impact reshapes the healthcare landscape, promising a future of precision medicine and enhanced healthcare delivery.
OpenGov Asia reported that MIT scientists harnessed artificial intelligence, particularly deep learning, to identify a compound group capable of battling drug-resistant bacteria. The researchers focused on MRSA, employing deep learning models to identify chemical structures associated with antimicrobial activity.
Their research showcased these compounds’ effectiveness in eradicating methicillin-resistant Staphylococcus aureus (MRSA), an organism causing over 10,000 deaths yearly in the United States.