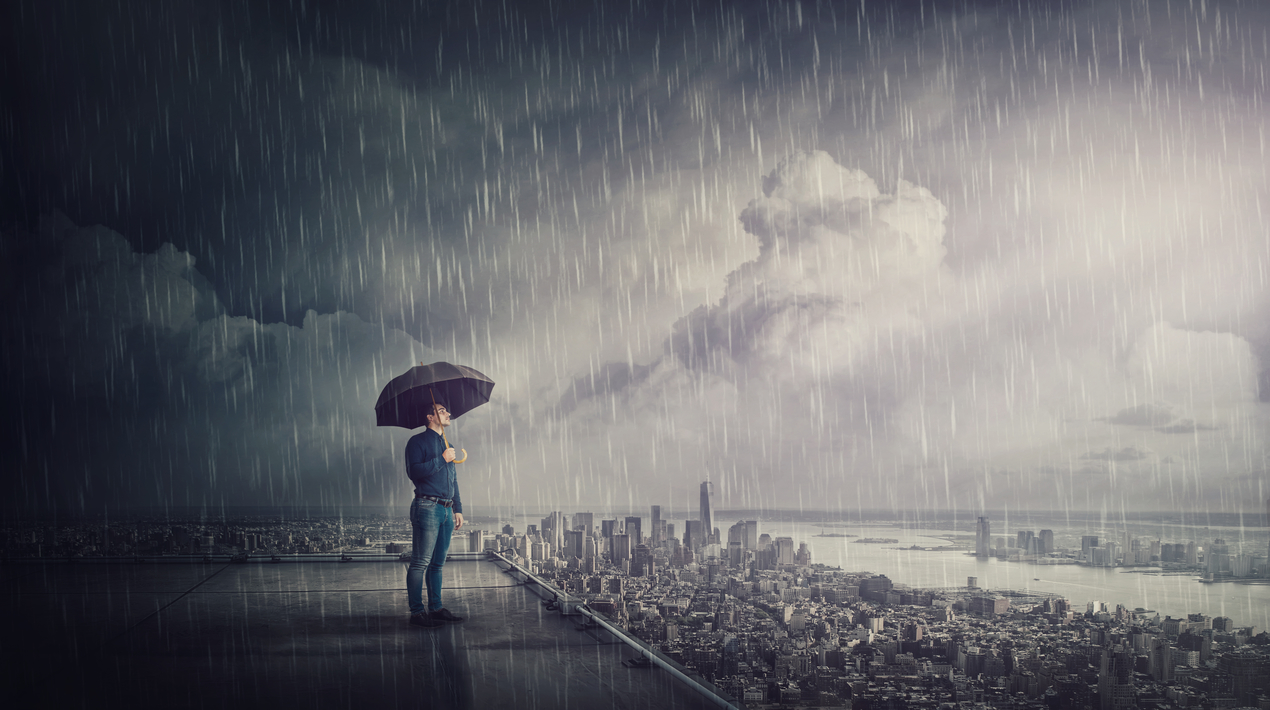
U.S. Researchers working on smart irrigation systems have developed a way to choose the most accurate weather forecast out of those offered in the week leading up to a given day. Every farmer would love to have a perfect weather forecast, but accurate forecasts are even more important to those who are embracing technology and in particular the Internet of Things (IoT).
In farming, IoT involves smart devices that talk to each other, to make recommendations such as when, where and how much to irrigate. That decision requires a lot of information, such as the needs of the particular crop, the current stage of its development, soil moisture and the weather.
IoT researcher
The researchers have been looking for ways to go beyond the standard weather predictions, such as the Bureau of Meteorology’s (BOM) seven-day forecast, to help farmers and their smart systems decide whether they need to irrigate today. Hence, the researchers have devised a hybrid system that shows real promise in improving the precision of rainfall forecasts.
People usually assume that the forecast closest to the day in question will be the most reliable, but that turned out not to be the case. So the researchers looked at ways to teach our artificial neural network to understand the relationships underlying all the data, to choose the best forecast. The team has developed a Hybrid Climate Learning Model (HCLM), which learns from a combination of the climate model data and the eventual answer to the question: is it going to rain tomorrow?
First, a probability-based network evaluates multiple forecasts for different rainfall patterns. Then a deep-learning neural network reprocesses the forecasts to produce a better prediction for the next day. Using high-quality processed data from the BOM rather than raw observations has helped the HCLM learn better.
The neural network examines the relationships between massive amounts of input data, processes it through many network layers, and learns from the mistakes made in earlier forecasts. The higher the quality of data, the better the network learns. When they then tested their system across that same range of climate zones, the hybrid model outperformed the BOM’s climate models and three other experimental systems, making the fewest errors in its forecasts.
The researchers are keen to stress that their work won’t be putting the BOM out of business. This work relies on their expertise, and the HCLM builds its rainfall predictions on the multiple forecasts produced by the BOM’s climate models. This model is the first to bring together the climate models, a probability network and a deep-learning neural network.
As reported by OpenGov Asia, to support weather prediction and the study of the Sun’s internal structure and dynamics. National Science Foundation’s (NSF’s) National Solar Observatory (NSO) has provided continual observational data of solar activity from a global network of six identical telescopes.
Recently, the NSO successfully transitioned the processing of these important observations of the Sun’s magnetic field and lower atmosphere to the operational control of the National Oceanic and Atmospheric Administration’s (NOAA’s) Space Weather Prediction Center (SWPC). This move will ensure reliable delivery of the data to the NOAA’s space weather forecasters who are the nation’s official civilian source for space weather watches, warnings, alerts, and forecasts.
NSO utilises advanced technological techniques developed by dedicated engineering teams to provide the highest quality data to the U.S. solar physics community. Scientists are conducting cutting edge research on understanding the fundamental processes that occur on the Sun, specifically how its active magnetic field influences solar activity, and using our understanding of solar behaviours to better inform the knowledge of other stars.