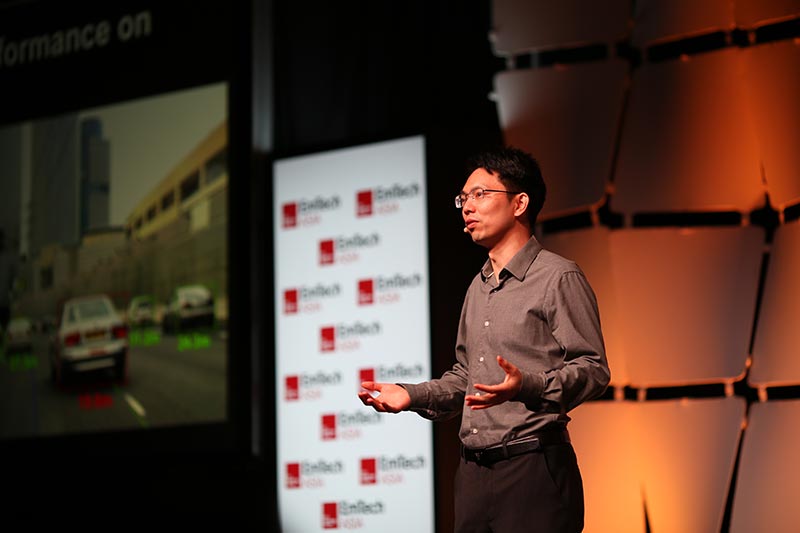
OpenGov spoke to Dr. Gang Wang on the sidelines of EmTech Asia 2017, where he was honoured as one of MIT Technology Review Innovators under 35.
Dr. Wang’s research interests include developing effective and efficient machine learning techniques which can advance the general artificial intelligence research and developing working computer vision systems and techniques.
He is a former Associate Professor (till March 2017) with the School of Electrical and Electronic Engineering at Nanyang Technological University (NTU), Singapore and an associate director of the Rapid-Rich Object Search (ROSE) Lab at NTU. The ROSE Lab is a joint collaboration between Nanyang Technological University, Singapore, and Peking University, China. He is currently Chief Scientist at Alibaba AI labs.
A team led by Dr. Wang achieved a top 5 ranking in the ImageNet challenge on scene classification in 2015 and 2016. The technologies invented by his group have been successfully licensed to 6 international and local companies.
Can you tell us about your work?
I am working on deep learning for the visual understanding problem. We want to make computers understand visuals like humans.
It is a hard problem. Scientists have been working on it since the 1960s. When I started thinking of this problem, I thought of learning from our brains. Our brains are very compact, low power consuming, also very intelligent. It is almost like there is a magic mechanism inside that has been developed during the long process of human evolution over a period of thousands of years.
What if we can leverage this mechanism to help the computers to learn? This has been tried a little bit but not very deeply. I worked with my students to go deep and find out what is the magic mechanism within our human brain. Then we try to model such a mechanism using deep neural networks.
The connection between the neurons in our brain is flexible and adaptive. When people try to recognise a cat versus say recognising cars, the connection inside the neurons might be slightly different. In the classic neural network methods, the connections are fixed between the different artificial neurons. I tried to make the connection between the neurons adaptive to the specific visual recognition test. We finally did it and we found that it resulted in significantly improved performance.
Where do you see your research going during the next 2-3 years?
I divide my research into two categories. One is more academic, more like fundamental research. In that, I will continue to push the boundary of brain-inspired deep learning algorithms. We also need to work closely with neuroscientists to try to understand our brains better, so that we can develop better algorithms.
The other side is applications. I am very interested in robotics. A general purpose robot needs to be able to sense the environment. Visual understanding is important for that. I want to transfer my visual understanding technology to robotics applications.
Where do computers stand now in visual recognition compared to humans?
We have made significant progress. Now computers can achieve very high accuracy in recognising thousand or more different categories.
So, right now, for this problem computers can do better than humans. They have the advantage of they can have more computing resources than humans. Humans cannot actually remember so many categories.
Can you give a basic explanation about how the understanding works?
There are two categories of understanding. The simpler one is for the purpose for navigation. In this case, you have to understand the 3D geometry, the environmental layout.
Suppose a robot is moving in this room, it needs to find what is the flow in order to navigate. To get to that chair, it needs to understand that there is something in the way. That is what we call a low level problem.
The second one is about more advanced applications. For example, once we have a humanoid robot, we ask the robot to give a cup of water. It must understand what is a cup and what is a cup of water. This is related to semantics. It has to understand object names, the meanings of object names and associate it with real world objects. This is human-like understanding.
Are we at the stage yet where the system will be able to understand that an object is a chair though it does not look like any chair it has been exposed to before?
Currently it is unlikely. We have to feed the computers with huge volumes of data with similar patterns to teach them what the object is. For the case you mentioned it requires the computer to have generalisation capability, which is very easy for humans.
What trends do you see in the areas of computer vision and deep learning?
I believe that the future is quite promising. We have achieved huge progress in many tasks such as vehicle detection for self-driving cars. And also, image classification for some online applications such as advertisement.
A lot of applications have been built based on deep learning technology. They are creating venues for commercialisation. Like in China, the banks can use the computers to verify the faces. They no longer require human workers. This saves them manpower costs.
Also, we are collecting more and more data because deep learning requires a lot of data. The more data we have, the better will be the performance of the technology.