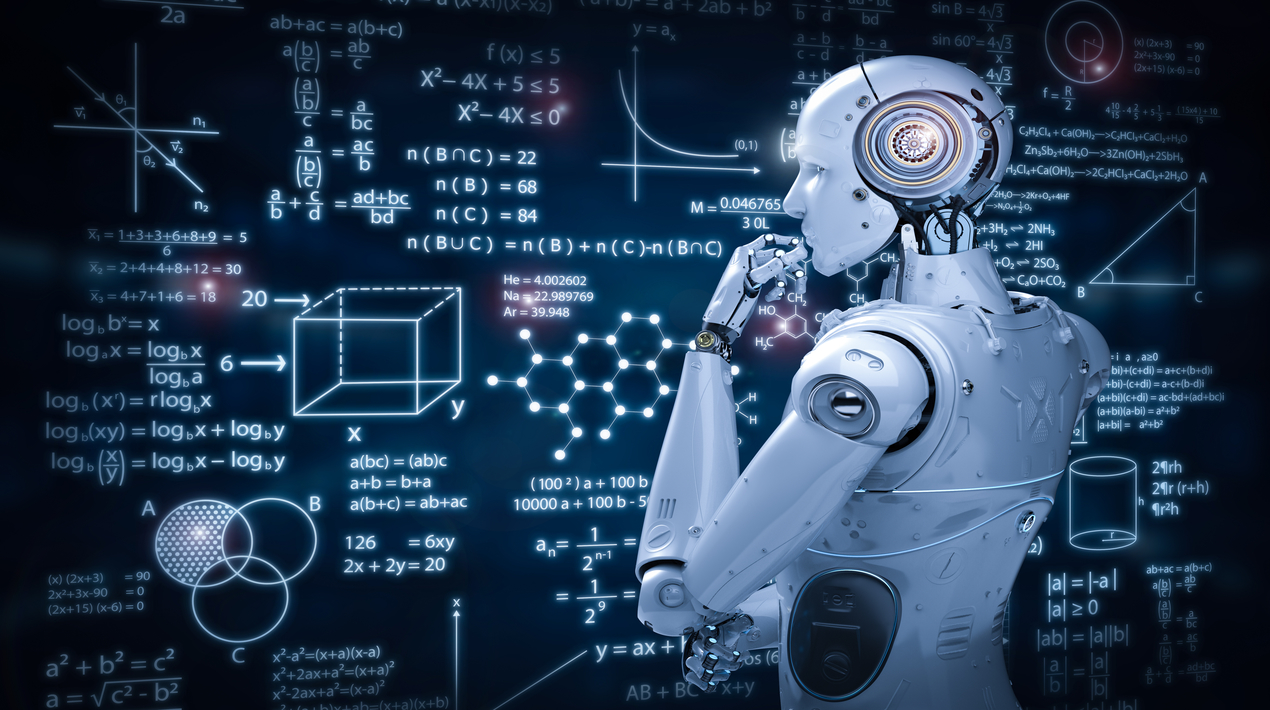
Researchers have developed an exoskeleton robot that uses a combination of lightweight material engineering and Artificial Intelligence to assist persons with mobility issues. A key feature of the new device is the technology that allows the skeleton to accurately predict the user’s intentions.
Robotic exoskeletons have the potential to help assist an elderly population. In essence, these are suits that people can put on to allow them to exert strength when their old bodies are unable of doing so. The fact that exoskeletons are often hefty and, if not correctly regulated, can behave as hindrances rather than aids has delayed their development. As a result, it’s critical to create exoskeletons that are both lightweight and capable of assisting rather than obstructing the user’s activities.
The current study has two primary components. First, the researchers created a light, carbon fibre-based lower-body exoskeleton that was linked to participants’ thighs and lower legs. The exoskeleton was designed with extremely back-drivable actuators to allow users to move freely even when the actuators were turned off. Furthermore, the research team looked at artificial intelligence to see if it could be used to forecast how the user will move.
They employed a technique known as PU-learning, or positive and unlabeled, to train the exoskeleton to accurately detect the user’s intentions based on muscular activity readings. By combining positively labelled data, which the machine knows is accurate, with other unlabeled data that could be either positive or negative, the PU-classification approach lets artificial intelligence learn from data that isn’t fully labelled.
Participants in the experiment stood up, crossed their legs, leaned forward and repositioned themselves on a chair, all of which can start in the same way. The exoskeleton employed machine learning to predict when they were attempting to stand up and then assisted them in doing so.
The experiment yielded positive results. In scenarios when user behaviour other than the goal sit-to-stand motion can occur, the results were better than conventional systems that employ fully labelled data, indicating that the technology could be generalised to other movements as well. The key finding of our study is that when programming a robot to help human movement, it’s critical to assume that humans will act in ways that aren’t predicted by the learning data.
Many exoskeletons are propelled by springs or motors, and if their joints are not aligned with the user’s, they might cause pain or harm. Researchers at the National Institute of Standards and Technology (NIST) devised a new assessment method to see if an exoskeleton and the person wearing it are moving smoothly and in sync to help manufacturers and users reduce these dangers.
The researchers describe an optical tracking system (OTS) that is similar to the motion capture techniques used by filmmakers to bring computer-generated characters to life. The OTS employs unique cameras that emit light and capture what is reflected back by spherical markers placed on target objects. The position of the labelled items in 3-D space is calculated by a computer. This method was used to track the movement of an exoskeleton and test items attached to its user, dubbed artefacts.
The goal of the current study was to record the motion of the knee, which is one of the body’s more simple joints. They built two prosthetic legs as testbeds to analyse the measurement uncertainty of their innovative approach. One used a store-bought prosthetic knee, while the other used a 3-D-printed knee that looked more like the actual thing. Exoskeletal limbs or test objects affixed to the body were represented by metal plates fastened to the legs with bungee cords.