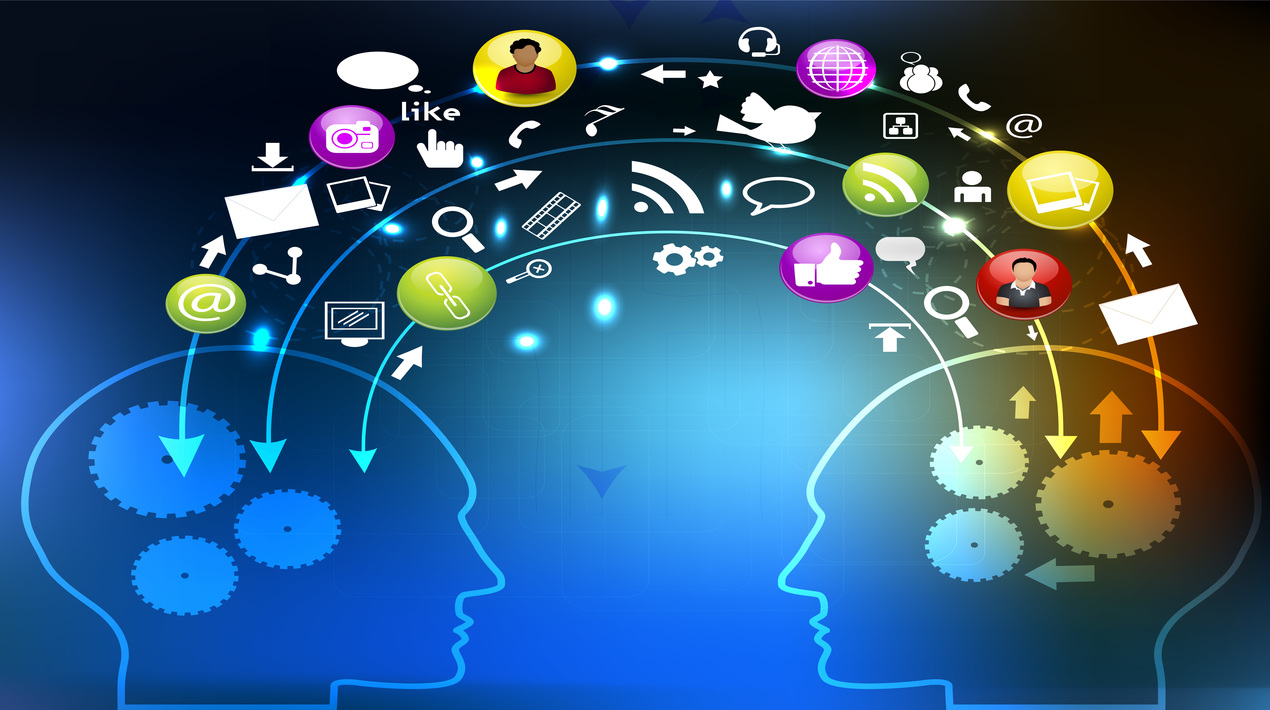
According to a study funded by the National Institutes of Health’s Brain Research Through Advancing Innovative Neurotechnologies (NIH BRAIN), a brain-computer interface (BCI) can restore communication to people who have lost the ability to move or speak. The BCI is designed specifically for people with spinal cord injuries and neurological disorders such as amyotrophic lateral sclerosis (ALS).
Researchers focused on the part of the brain that is responsible for fine movement and recorded the signals generated when the participant attempted to write individual letters by hand. In doing so, the participant, who is paralysed from the neck down following a spinal cord injury, trained a machine-learning computer algorithm to identify neural patterns representing individual letters.
While demonstrated as a proof of concept in one patient so far, this system appears to be more accurate and more efficient than existing communication BCIs. This BCI could help people with paralysis rapidly type without needing to use their hands.
When a person becomes paralysed due to spinal cord injury, the part of the brain that controls movement still works. This means that, while the participant could not move his hand or arm to write, his brain still produced similar signals related to the intended movement. Similar BCI systems have been developed to restore motor function through devices like robotic arms.
After a series of training sessions, the BCI’s computer algorithms learned how to recognise neural patterns corresponding to individual letters. It allows the participant to “write” new sentences that had not been printed out before, with the computer displaying the letters in real-time. This system provides a level of flexibility that is crucial to restoring communication.
With this BCI, their study participant, whose hand was paralysed from spinal cord injury, achieved typing speeds of 90 characters per minute with 94.1% raw accuracy online, and greater than 99% accuracy offline with a general-purpose autocorrect. These typing speeds exceed those reported for any other BCI and are comparable to typical smartphone typing speeds of individuals in the age group of our participant (115 characters per minute).
NIH BRAIN Initiative states that this study represents an important milestone in the development of BCIs and machine learning technologies on how the human brain controls communication processes. This knowledge is providing a critical foundation for improving the lives of others with neurological injuries and disorders. Therefore, the results open a new approach for BCIs and demonstrate the feasibility of accurately decoding rapid, dexterous movements years after paralysis.
The development of this BCI is in line with NIH’s mission to seek fundamental knowledge about the nature and behaviour of living systems and the application of that knowledge to enhance health, lengthen life, and reduce illness and disability. NIF has also funded another study that utilises technology to reduce illness and disability.
As reported by OpenGov Asia, a mobile app can distinguish toddlers with autism spectrum disorder (ASD) from typically developing toddlers based on their eye movements. They observe the toddlers while they are watching videos during their pediatric visit. This is because toddlers with ASD have distinctive eye-gaze patterns and reduced attention to social stimuli. They can also barely coordinate gaze with speech sounds.
The study found that the app deployed on an iPhone or iPad reliably measures both known and new gaze biomarkers that distinguished toddlers with ASD vs typical development. This method may have the potential for developing scalable autism screening tools. It can possibly apply to a natural environment and enable data sets compliant to machine learning.
Researchers record the children’s gaze patterns with the device’s camera and measure them using computer vision and machine learning analysis. Children with ASD were less likely than typically developing children to focus on social cues and visually track the conversations in the videos.
In the future, the mobile app can screen infants and toddlers for ASD and refer them for early intervention when chances for treatment success are greatest. However, equipment used for visual tracking is expensive and requires specially trained personnel, limiting its use outside of laboratory settings.