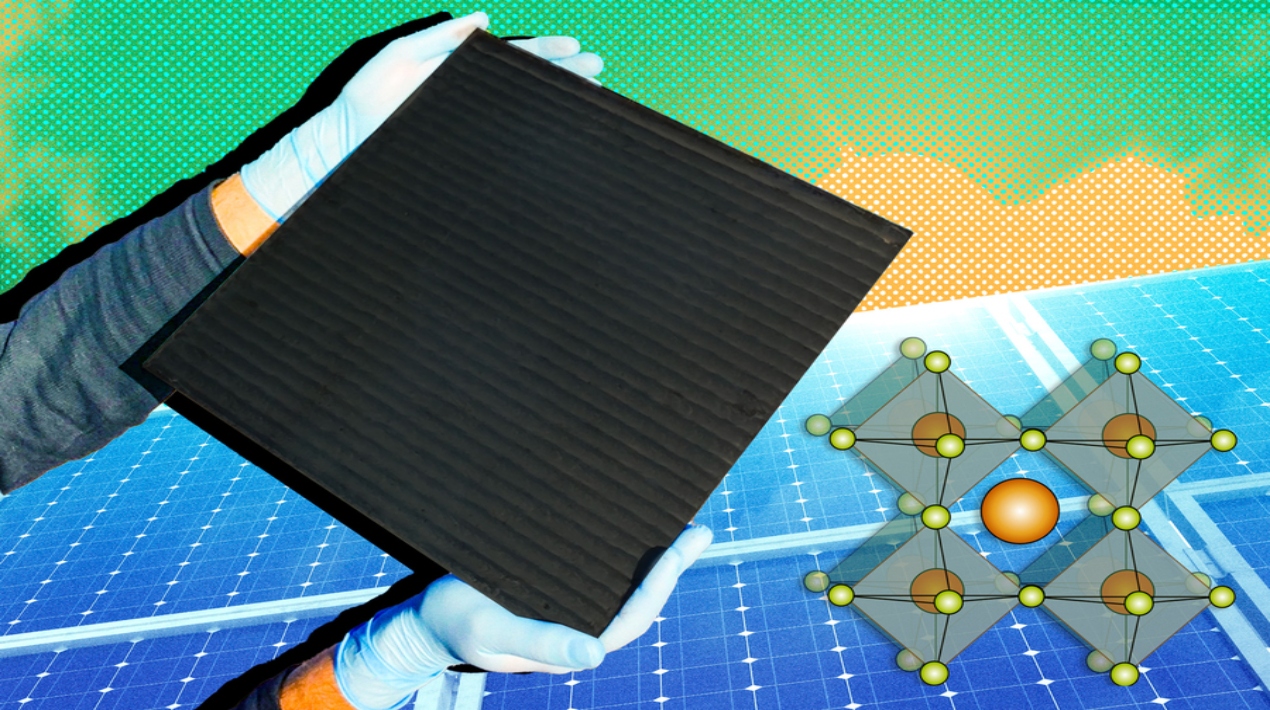
The most likely replacement for present silicon-based solar photovoltaics is a family of materials called perovskites. They have the potential for panels that are far lighter and thinner, which might be produced at ultra-high through placed at room temperature rather than at hundreds of degrees, and which are also less expensive and simpler to carry and install.
Researchers are working on encapsulating the perovskite in various protective materials to protect it from exposure to air and moisture. They are also researching the precise mechanisms that cause that degradation in the hope of developing formulations or treatments that are more inherently robust. A key discovery is that the breakdown is largely due to a process known as autocatalysis.
With this, researchers have developed a machine-learning approach that can significantly streamline the search for promising new candidate compositions for perovskites. This process is analogous to seeking a needle in a haystack. This novel strategy could expedite the creation of new alternatives.
While most machine-learning systems utilise raw data such as measurements of the electrical and other properties of test samples, they typically do not incorporate human experiences such as qualitative observations made by experimenters of the visual and other properties of test samples, or information from other experiments.
Consequently, the researchers devised a mechanism to include such external information into the machine learning model, utilising a Bayesian Optimisation-based probability component. This new system based on an innovative approach to machine learning could accelerate the development of optimised production methods and contribute to the realisation of the next generation of solar power.
This advanced technology, developed by the Massachusetts Institute of Technology and Stanford researchers enables the incorporation of data from previous trials and information based on the personal observations of experienced workers into the machine learning process.
It has improved the accuracy of the results and has already led to the production of perovskite cells with an energy conversion efficiency of 18.5 per cent, which is now viable for the current market.
On the other hand, manufacturing perovskite-based solar cells necessitate the simultaneous optimisation of a dozen or more factors, and that’s just one of many possible manufacturing methods.
Perovskites have a high tolerance for structural flaws, which is one of their major advantages. It can function well even with numerous imperfections and impurities, unlike silicon, which requires extremely high purity to function well in electronic devices.
However, at least a dozen variables may impact the outcome of this procedure. It is impossible to evaluate every conceivable combination of these factors through testing; hence, machine learning was required to lead the experimental procedure.
Thus, most of the existing research on machine-learning-driven perovskite PV fabrication focuses on spin-coating, a lab-scale technique and only a few organisations have the expertise in both engineering and computation to drive such advances.
Furthermore, perovskites continue to show enormous potential, and several businesses are now preparing to commence commercial manufacturing, but durability remains their greatest challenge. Perovskites degrade considerably faster than silicon solar panels, which preserve up to 90 per cent of their power production after 25 years.
Initial samples lasted only a few hours, then weeks or months, but current formulations have useful lives of up to a few years, making them acceptable for applications where durability is not important. The primary goal of the researchers was to accelerate the process, so it required less time, fewer experiments, and fewer human hours to develop something that is usable right away, for free, for the industry.