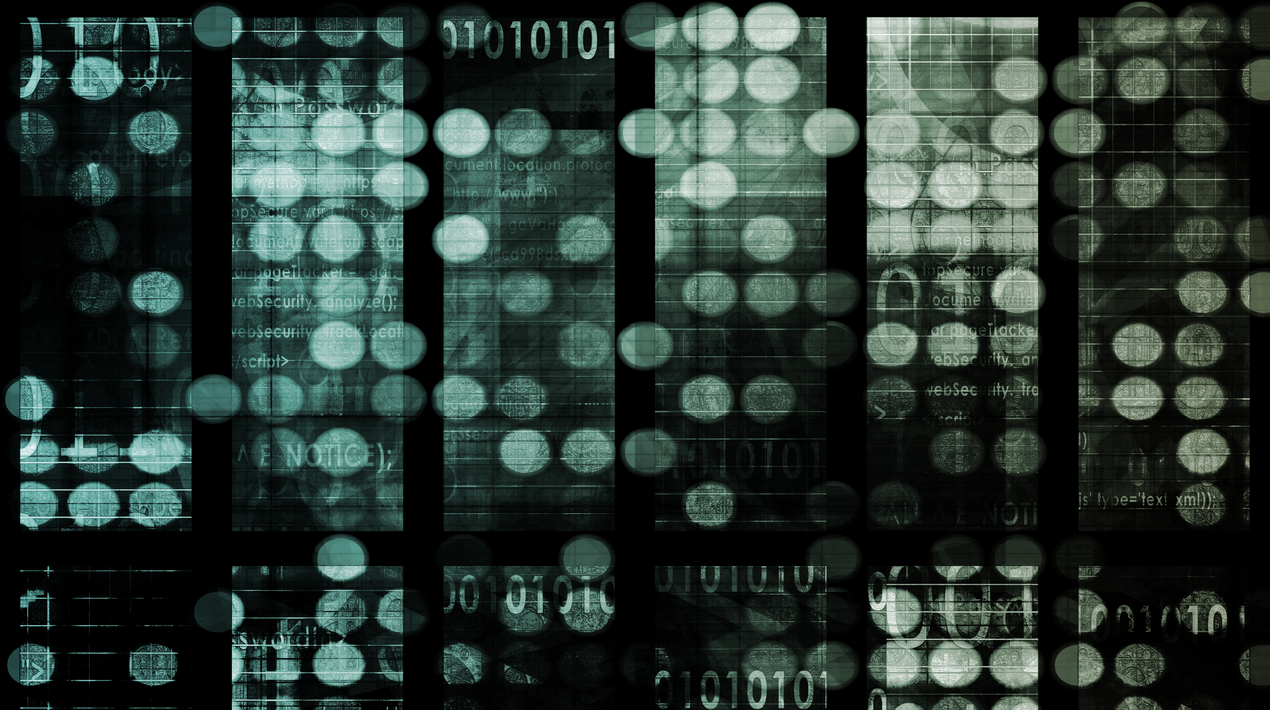
Researchers from the Brookhaven National Laboratory of the US Department of Energy have developed a novel machine learning (ML) framework that can pinpoint which phases of a multistep chemical reaction can be adjusted to boost productivity.
The method could aid in the development of catalysts, which are chemical “dealmakers” that speed up reactions. It was created to investigate the conversion of carbon monoxide (CO) to methanol using a copper-based catalyst.
The reaction is made up of seven relatively simple elementary steps and was used as an example by the researchers in their ML framework method. The goal was to determine which elementary step or subset of steps in the reaction network controls the catalytic activity.
Traditionally, researchers attempting to improve such a reaction would calculate how changing each activation barrier one at a time might affect the overall production rate. This type of analysis could determine which steps were “rate-limiting” and which steps determined reaction selectivity—that is, whether the reactants proceeded to the desired product or via an alternate pathway to an undesirable by-product.
The new machine learning framework is intended to improve these estimates so that scientists can more accurately predict how catalysts will affect reaction mechanisms and chemical output. The scientists began by collecting data to train their machine learning model. The data set was created using “density functional theory” (DFT) calculations of the activation energy required to transform one atom arrangement to the next over the course of the reaction’s seven steps.
The scientists then used computer simulations to see what would happen if they changed all seven activation barriers at the same time – some going up, some going down, some individually, and come in pairs.
They generated a comprehensive dataset of 500 data points by simulating variations in 28 “descriptors,” which included the activation energies for the seven steps as well as pairs of steps changing two at a time. This dataset forecasted how individual and pairwise tweaks would affect methanol production. The model then ranked the 28 descriptors in terms of their significance in driving methanol output.
The scientists retrained the ML model using only the six “active” descriptors after identifying the important descriptors. Based solely on DFT calculations for those six parameters, this improved ML model was able to predict catalytic activity.
The model, according to the team, can also be used to screen catalysts. The model predicts a maximum methanol production rate if it can design a catalyst that improves the value of the six active descriptors.
When the researchers compared their model predictions to the experimental performance of their catalyst—as well as the performance of alloys of various metals with copper—the predictions matched the experimental findings. Comparisons of the ML approach to the previous method for predicting alloy performance revealed that the ML method was far superior.
The data also revealed a lot about how changes in energy barriers might affect the reaction mechanism. The interaction of the various steps of the reaction was of particular interest—and importance. For example, the data showed that lowering the energy barrier in the rate-limiting step alone would not improve methanol production in some cases. However, increasing methanol output by adjusting the energy barrier of a step earlier in the reaction network while keeping the activation energy of the rate-limiting step within an ideal range.